From pixels to melodies
: image based playlist generation




Product Design
ML Integration
Personalization
Machine Learning-based, context-aware music playlist generation for personalized, matching playlist
Why is finding a playlist feel like a research project?
As a music lover, I found myself endlessly scrolling searching for the right song. It became more about finding right keywords and guess work than vibes. That’s when I imagined: What if we could choose music the way we capture memories? Powered by machine learning, Snapmusic found the new way of music discovery to make experience more intuitive and emotional.
Role
UX designer
Timeline
May - July 2023
Remastered 2025
Project
Personal Project
tools
Figma / Figjam
Adobe Photoshop, Illustrator
Overview
Problem
When recommendations lack context, miss personal taste, or fail to support group dynamics, users spend more time searching than enjoying.
Search-focused playlists in current music apps


Solution
Leveraging context awareness and visual cues to create personalized playlists
Snapmusic tackles these gaps by turning the photos and memories people already share into instantly context‑aware, taste‑aware, and group‑inclusive soundtracks—eliminating scroll fatigue and making every playlist feel “just right.”
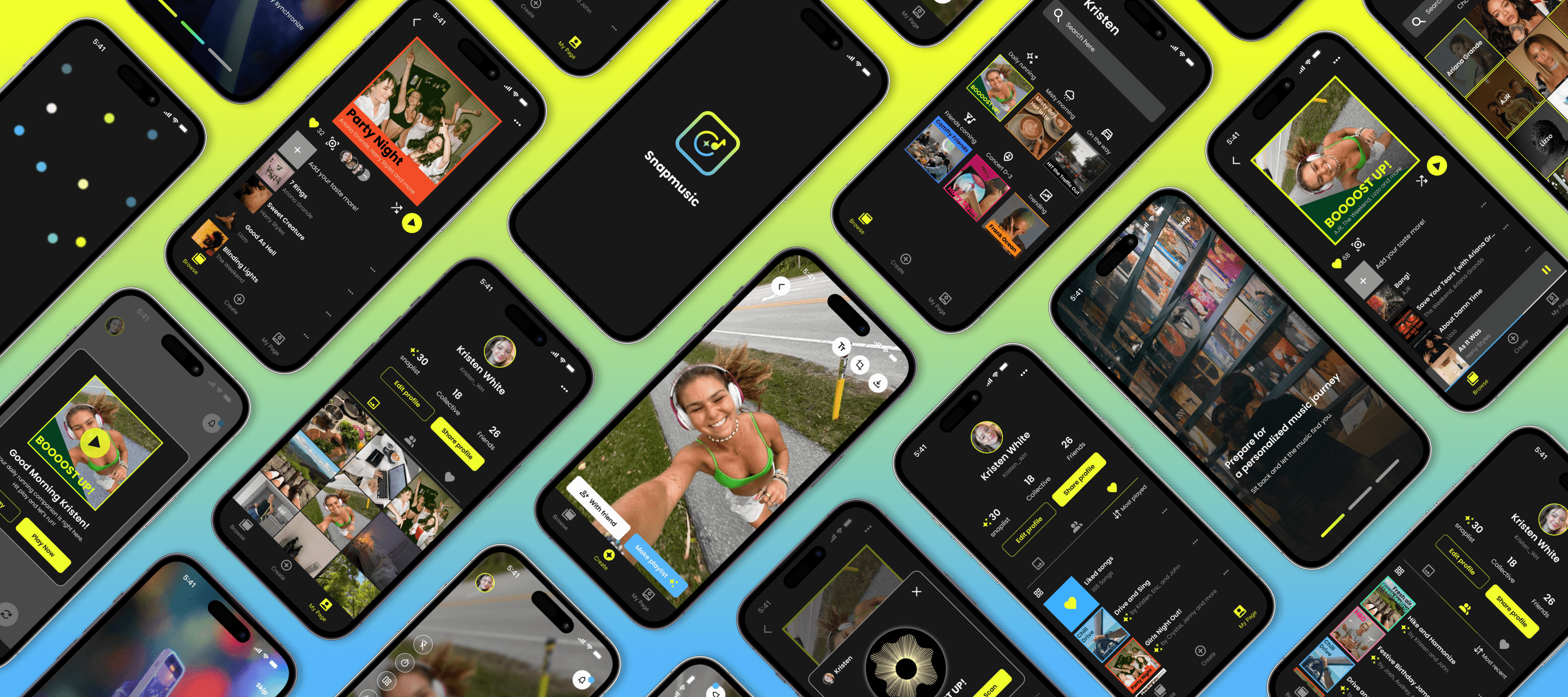
Outcome
Key features that solve choice overload, social pressure, and taste-mismatch
Snap and create personalized playlist in a second
Upload a photo and let the system instantly read the scene to spin a perfectly‑matched playlist to the moment.
Relieve pressure and vibe with group
Share your “Snaplists,” invite friends to add photos or tracks, and let the app blend everyone’s taste so no one has to play DJ alone.
Present magical coincidence
By reading time, location, and past listens, Snapmusic surfaces custom playlists at the exact moment you want them even before you even ask.
Design Process

Research 1
Research Goal & Matching Research Methods
▶︎ Competitive Analysis
▶︎ Quantitive Research through Survey
▶︎ Technology Exploration
How did we arrive at this problem space?
Survey Discovery
To hear from a broader range of users, I conducted a survey with 40 frequent music app users. The results revealed that many struggle when choosing a playlist, highlighting key pain points and opportunities to improve the overall music selection experience.
What exactly are the problems of current platforms?
Competitive Analysis
Survey participants named Spotify, Youtube Music, and Apple Music as their top three music platforms. My analysis mapped each service's uniqueness, strengths, and pain points. While each stands out in different areas, they all facing with the same core issue:
Problem of balancing personalization, exploration, and cluttered mix of contents.
How did I narrow down?
Problem Discovery with Three Pain Points
Just as music preferences differ, their experiences with music platforms also vary but all of the users wanted to choose matching playlist easily and intuitively. The survey and competitive analysis revealed the real challenge isn’t just finding any playlist, but it’s making the right choice at the right moment without stress.
Time-Consuming Trial and Error
Because playlist search is keyword-based and emotionally vague, users have to click through multiple options just to find one that feels right.
Decision Anxiety
When under time pressure or trying to choose music for a group, users feel overwhelmed and second-guess their selections.
Discovery Bubble
Despite endless content, users often feel stuck in a loop of popular playlists, with limited tools to find music that feels new, personal, or unexpected.
Explore Metaphors for Music Discovery
Understanding the problem of endless browsing in thousands of options, I wanted to explore new way of music discovery. Because music shouldn't be a search task like research project. To develop a core feature for an accessible and personalized experience, I explored various metaphors to translate abstract emotions into visualized data.
Wearable Smart Ring
Dynamic biometric data is super-personalized and hands-free input
Limited scope and lower accessibility by physical artifact
Natural Language Input
Personalization and accommodation
Familiar to use
Difficulty describing every contexts
Visual Cue (Image)
Convey emotion, activity, and personal context instinctively
Access to human factors and physical environment context
Use of explicit metaphor -> lower the awareness mismatch
Emoji Selection
Quick selection in fun and intuitive way of playful interaction
Ambiguous & different interpretation error
Result: Through quick brainstorming sessions, I identified the most effective approach for generating playlists that resonate with users' moods and contexts so I chose to utilize visual cue (image) for metaphor because it conveys mood and activity the most contextually.
Challenge
Design Challenge
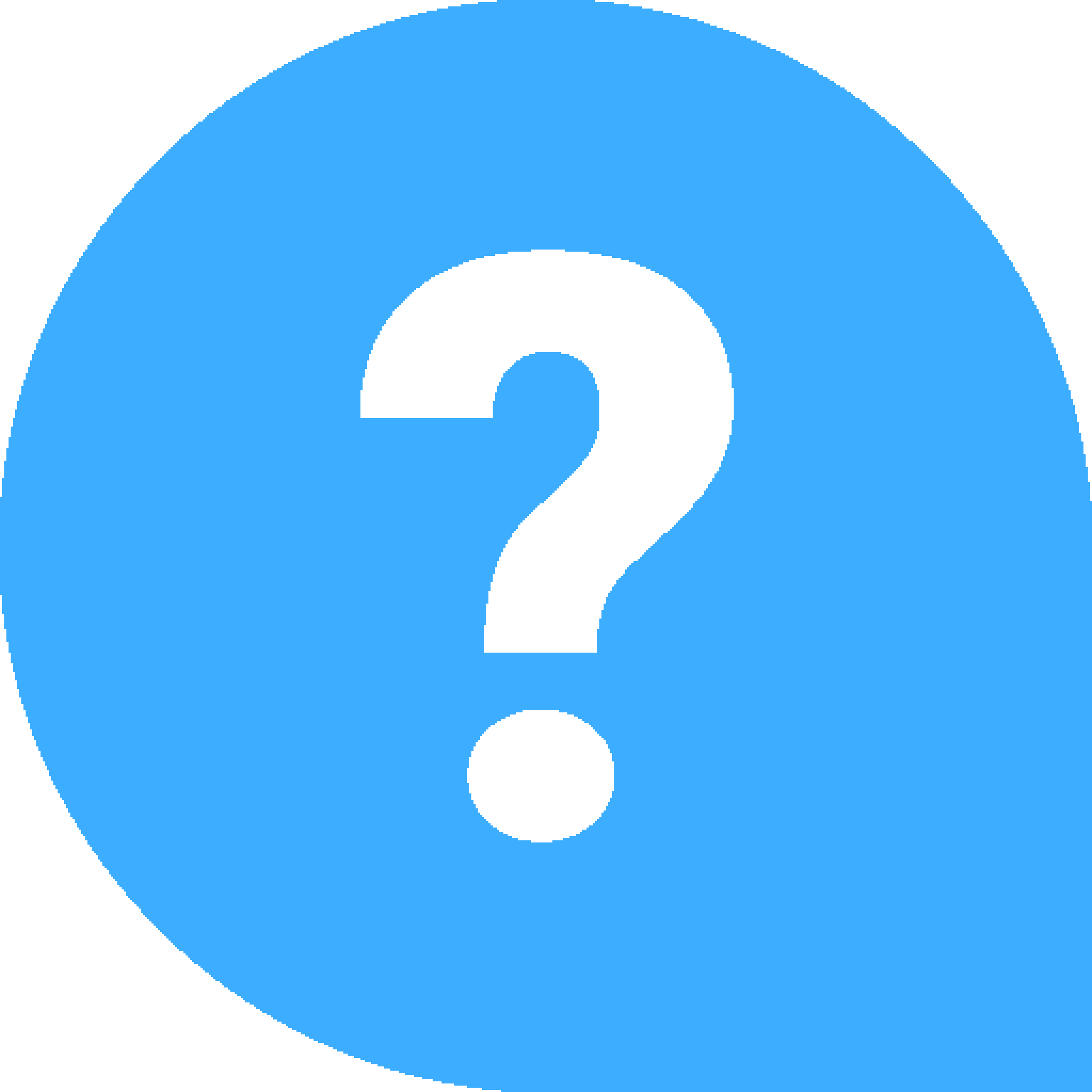
How might we utilize visual cues and leverage context-based technologies to simplify music discovery and create personalized playlists?
Research 2
Understanding Computing Technology
To check feasibility of the concept, I researched computing recognition technologies by myself and asked to two software engineers to explore how they could be applied to my concept of recognizing context from images. Even though it was my independent study, it was crucial to understand the system to design interactions and evaluate time for understanding the context and transforming into a playlist in real setting.
How does the technology execution process work?
How Snapmusic is different than others?
Following the Hick's Law, as a user spends more time making a decision, the complexity of choices also increases. Image-based Machine Learning can reduce the cognitive load of choosing a playlist.


Ideation
Target User Persona & Storyboard
Through analyzing user research, I developed two main user personas that best embodied target audience's needs and pain points. After then, I turned Eric's scenario into a storyboard.
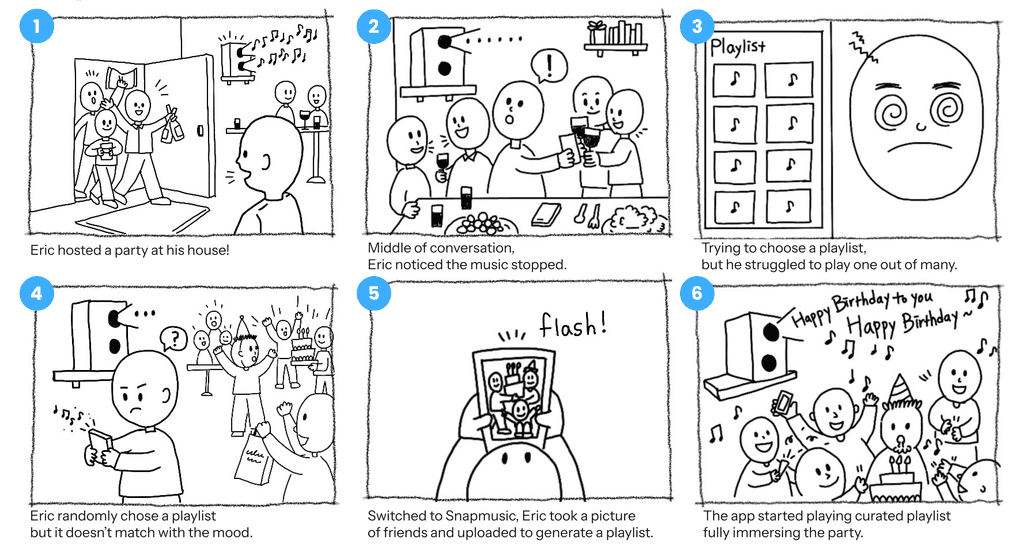
Wireframe
Wireframe and Flow Design

Design Iterations
Home Design Iteration and Improvements
I started by drawing inspiration from music apps on market, displaying playlists in the Home with varying sizes of cards for visual hierarchy. After two mentorship sessions, I realized my initial designs lacked a context-based approach. I learned the importance of intuitive interface design and simplified the user flow to bring users closer to action in fewer steps.
Design Issues
⏶ The list views contribute to cognitive overload rather than simplifying the process, making it less convenient for users.
⏶ Fails to clearly highlight its unique feature, which undermines the app's value proposition making uncertain about its purpose.
Improvements
● Removed lists and simplifies navigation and focuses user attention on its feature, reducing selections to decide.
● By replacing the 'Home' page with a 'Create' page, it curates users directly to the camera interface, emphasizing the app’s core feature of creating playlists from images.
Design Solution
Introducing Snapmusic!




Design Concept
Style Guide
I created a comprehensive design system for unified brand consistency by organizing standardized design elements, components, and guidelines that illustrates what was used in the final design and logo design.
Poster presentation at EXPO 2025, University of Michigan
How the judges have commented on Snapmusic?
"I could easily see companies such as Spotify or Apple Music being extremely interested in an idea such as this (I mean, even I would love to use Snapmusic)."
"I want to express an appreciation for Biju's hard work that she put into this independent project. Her presentation/pitch flows well, and it is concise. Overall, a great effort made by Biju!"
"The explanation of the underlying functionality and inputs paints a bigger picture beyond simply being a visual AI input, which is exactly the right direction to move in (and such a great idea to latch onto - it feels very feasible)."
"Such a cool idea. Using image would be a good companion to a more traditional, music taste driven approach. BTW, you have a great portfolio and are clearly super creative-- don't be afraid to flex that when you present!"
Lessons Learned
Takeaways
Leveraging Technology for Effective Solutions
Prior to starting the project, I had little knowledge of how AI, Machine Learning, and Computer Vision work. Through studying these technologies, I not only guided the design process effectively but also realized how my hypothetical idea could transform into reality. This experience highlighted the importance of research and knowledge in expanding creative possibilities.
Designing for Context Awareness
I learned the importance of designing for context awareness by continuously iterating on how users interact with systems in real-world scenarios. Understanding how environmental, behavioral, and situational factors influence user needs helped me refine interactions to feel more natural, intuitive, and responsive.
Next Steps
Implement Advanced Personalization
Currently, the research lacks in-depth user behavior analysis, which may lead to misunderstandings about application usage. If possible, I aim to address this by conducting usability testing to explore additional metadata, such as user preferences, social trends, and fitness activity data.
Transform SnapMusic into Reality
If I had more time, I would focus on strengthening SnapMusic’s technological foundation by collaborating with engineers to develop and refine playlist generation algorithms.